As customer service and support technology leaders, have you ever questioned how your customers interact with your brand, how they perceive your product or service, and how they talk about it? Conversation Analytics (CA) platforms are expanding to answer these questions by covering both voice and text channels. Leveraging Generative AI, these platforms help improve agent productivity through features like automated call disposition and call summarization. According to Gartner, by 2027, 75% of service and support organizations will have reduced human agent QA teams by over 50% due to the application of CA platform technologies. This article provides a comprehensive overview of what conversation analytics is, how it works, common uses, its advantages, and implementation strategies.
Table of Contents
ToggleWhat is Conversation Analytics?
Conversation Analytics (CA) refers to the use of AI and Machine Learning technologies to analyze customer interactions across multiple channels, including voice calls, live chat, email, and social media. CA is also known by terms such as speech analytics, conversation intelligence, and interaction analytics.
These platforms are designed to support customer service and support functions by improving the experiences of both customers and agents, detecting customer sentiment, flagging at-risk customers, monitoring compliance, and offering actionable insights through real-time agent support tools.
Why is Analyzing Customer Conversations Important?
Understanding customer interactions provides a foundation for improving support services, training agents, and aligning operations with customer needs. Below, we examine the specific reasons why analyzing conversations can lead to more efficient and responsive customer service models.
Improving Customer and Agent Experience
Analyzing conversations allows organizations to identify recurring issues, misunderstandings, or procedural inefficiencies. These insights can lead to improvements in call handling procedures, reduce customer wait times, and streamline agent workflows. With clearer understanding of pain points, companies can adjust processes and protocols to reduce friction.
Identifying Customer Sentiment and Risk
CA platforms can detect emotion and sentiment using NLP and machine learning. Recognizing negative sentiment early allows organizations to intervene proactively before customer dissatisfaction escalates. It also helps in identifying loyal or high-value customers based on interaction tone and language.
Ensuring Compliance with Policies
Compliance with internal guidelines and external regulations such as PDPA or GDPR is non-negotiable. Conversation analytics platforms automatically monitor whether agents follow scripts, avoid prohibited language, and stay within regulatory frameworks. This reduces compliance risk and improves overall audit readiness.
Supporting Agent Coaching and Development
Using conversation analysis, managers can identify performance gaps and training opportunities. For instance, metrics related to script adherence, response time, or resolution effectiveness provide data-driven bases for coaching sessions. This supports continuous development and contributes to higher agent satisfaction and performance.
Conversation Analytics vs. Traditional Analytics
The table below highlights the key differences between conversational analytics and traditional analytics approaches:
Aspect | Conversational Analytics | Traditional Analytics |
Data Source | Real-time customer-agent interactions on voice, chat, email, and social media | Historical structured data from CRM systems, databases, and call records |
Scope | Focused on analyzing live and historical conversations | Broad analysis across marketing, sales, and support data |
Real-time | Yes, supports immediate actions | No, typically retrospective |
Metrics | CSAT, AHT, sentiment, agent performance | Revenue, churn rate, campaign effectiveness |
Decision-Making | Service quality and training decisions | Business strategy and forecasting decisions |
Use Cases | Agent coaching, sentiment detection, issue resolution | Website performance, sales trend analysis |
Technology | AI, NLP, ML for unstructured data | Traditional BI tools and SQL-based systems |
How Does Conversation Analytics Work?
Conversation analytics involves multiple technical processes that work together to extract actionable insights from conversations. Let’s explore the four major stages involved in conversation analytics.
Data Cleanup
The first stage involves cleansing raw data by removing irrelevant elements such as filler words, special characters, and inconsistent grammar or punctuation. This ensures uniformity and prepares the text for accurate analysis. This phase is crucial for making noisy, unstructured data understandable by AI models.
Understanding Language
Natural Language Processing (NLP) plays a vital role in interpreting the meaning of words, phrases, and sentences. NLP helps contextualize expressions and understands intent, tone, and sentiment within the conversation. It allows platforms to recognize domain-specific terminology and nuances in different languages or dialects.
Finding Patterns
Once the language is processed, machine learning algorithms detect recurring patterns such as commonly asked questions, repeated complaints, or frequently used keywords. Over time, these algorithms become more effective by learning from vast datasets. This supports trend detection, escalation prediction, and proactive issue resolution.
Reporting Insights
Finally, the system visualizes insights through dashboards, alerts, or reports. These summaries point out service gaps, agent strengths, and areas where customer experience can be improved. This visualization layer enables informed decision-making for supervisors and business leaders.
5 Common Uses of Conversation Analytics
Conversation analytics platforms today are equipped with advanced features that go beyond simple call transcription. Under this section, we explore five widely adopted use cases supported by modern conversation analytics systems.
1. Multilingual Speech-to-Text Transcription
Advanced conversation analytics tools use Automatic Speech Recognition (ASR) to convert voice interactions into accurate text in real-time. These systems support over 50 languages, including English, Chinese, Thai, Vietnamese, Bahasa Malaysia, and Bahasa Indonesia, making it possible to serve a multilingual customer base. This feature ensures precise transcription for consistent data analysis across regions.
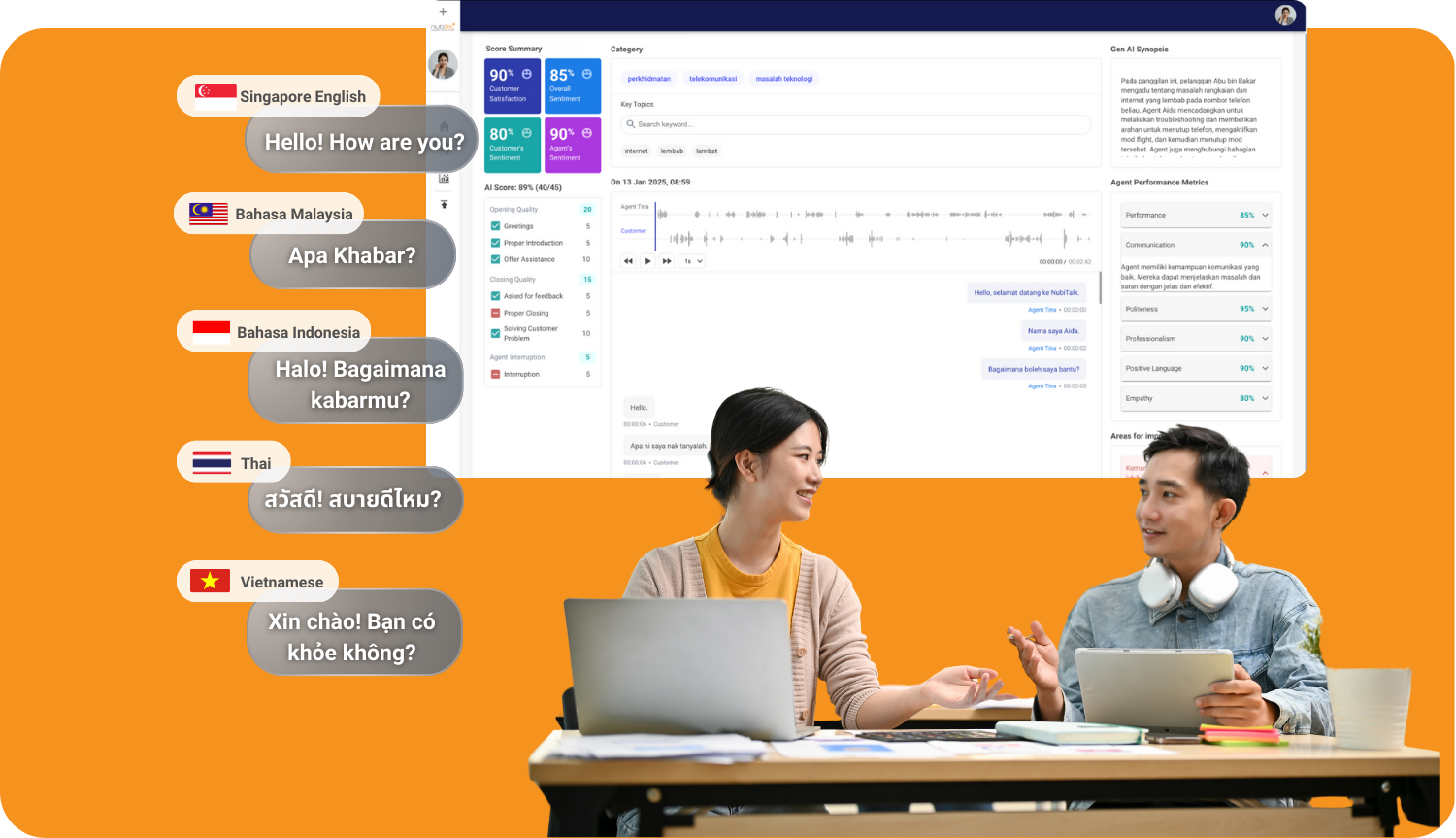
2. Sentiment and CSAT Analysis
AI models analyze emotional tone and sentiment within each conversation to determine if interactions are positive, neutral, or negative. Some platforms go further by automatically estimating Customer Satisfaction (CSAT) scores based on verbal cues and word choices.
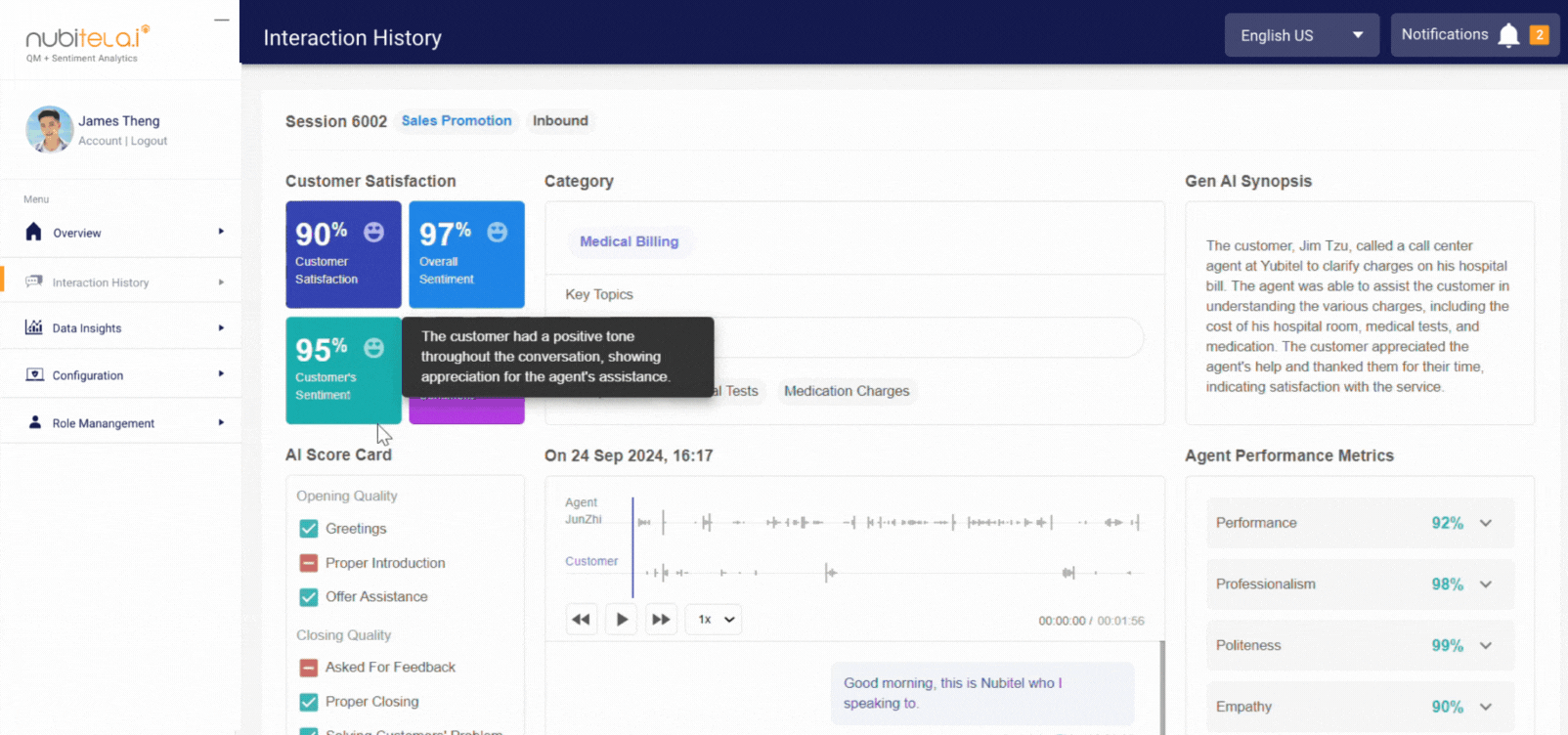
These insights help identify dissatisfied customers, training opportunities, and areas that require attention.
3. AI-Generated Summaries
Generative AI is increasingly used to produce concise, structured summaries of customer interactions. These summaries capture key discussion points, resolutions, and follow-up items.
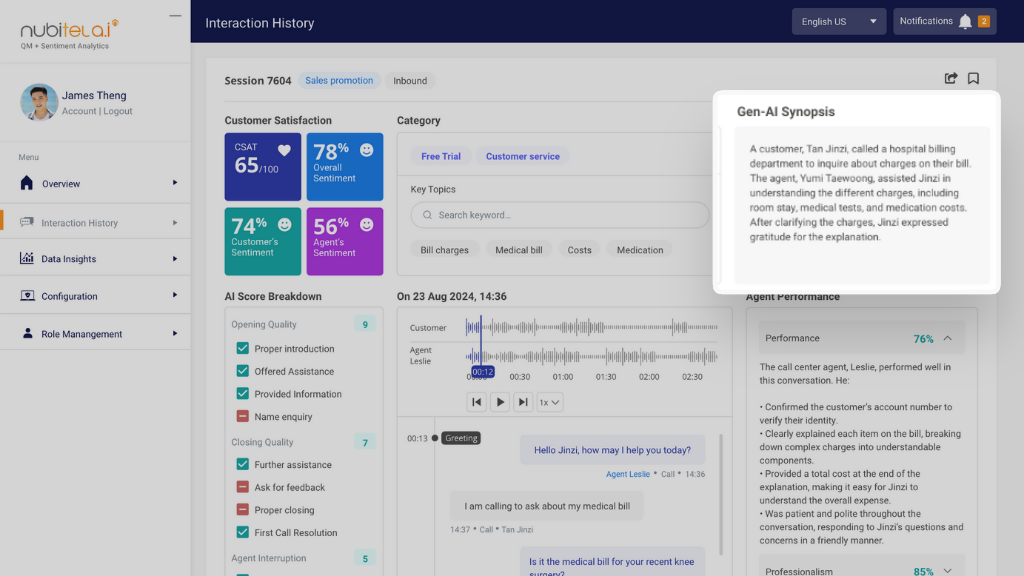
They support time-saving in manual QA reviews and make it easier for agents and supervisors to understand interaction outcomes at a glance.
4. Agent Performance Monitoring
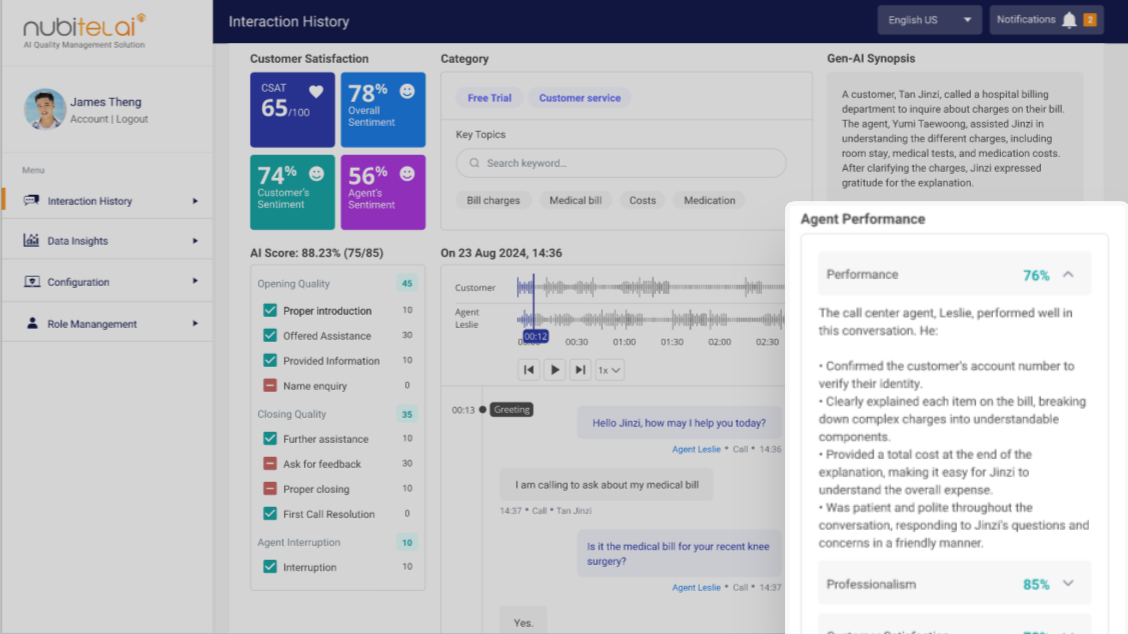
Conversation analytics tools assess agent behavior by tracking metrics such as talk ratio, interruptions, empathy usage, and script compliance. Quality scores are automatically generated to highlight strengths and pinpoint areas for improvement. These insights enable targeted coaching and improve overall service quality.
5. Topic and Keyword Detection
AI models detect frequently mentioned topics, phrases, or keywords across thousands of interactions. These patterns inform next-best-action strategies, guide product or service updates, and uncover emerging issues. With accurate topic mapping, teams can respond faster to evolving customer needs.
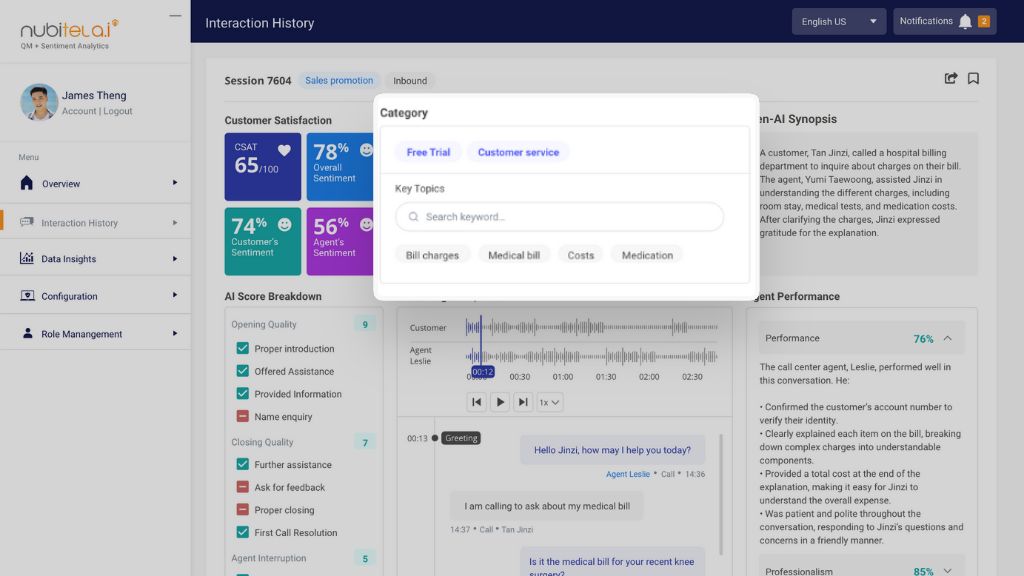
5 Key Benefits of Conversation Analytics
Conversation analytics unlocks a wide range of business and operational advantages that enhance service quality, agent efficiency, and strategic alignment.
-
Better Decision-Making with Data-Driven Insights
Conversation analytics provides leaders with data-rich insights into real-time customer feedback and agent performance. These insights can guide strategic decisions around training, process optimization, and service innovation. For example, if analysis reveals frequent product-related queries, the knowledge base can be updated or product training reinforced.
-
Operational Efficiency
By identifying root causes of repeated customer issues, companies can streamline internal processes, reduce call handling time, and optimize workforce allocation. Conversation analytics reduces the need for manual QA, allowing human supervisors to focus on higher-value tasks like agent coaching and complex escalations.
-
Enhanced Customer Experience
Real-time feedback from conversations helps companies personalize interactions and resolve issues faster. Proactive intervention based on sentiment detection or escalation alerts improves overall customer satisfaction. Over time, this contributes to increased customer loyalty and retention.
-
Smarter Agent Training and Coaching
Data from conversation analytics helps managers understand individual agent strengths and weaknesses. Personalized coaching plans based on real call examples lead to more effective skill development. Additionally, agents can learn from top-performing peers by reviewing annotated transcripts.
-
Stronger Compliance and Risk Management
CA platforms help ensure agents adhere to compliance scripts, use appropriate language, and follow customer verification protocols. Automated compliance checks reduce audit preparation time and mitigate regulatory risk.
Challenges in Implementing Conversation Analytics
While the benefits are substantial, organizations should be mindful of the following challenges when implementing conversation analytics solutions.
Data Privacy and Security
Handling large volumes of customer conversation data requires strict compliance with data protection laws such as PDPA (Singapore), GDPR (Europe), or other local regulations. Data anonymization, encryption, and access controls must be enforced to protect sensitive information.
Integration with Legacy Systems
Some organizations may find it difficult to integrate conversation analytics platforms with older CRM or telephony systems. Ensuring compatibility and smooth data exchange is crucial for generating unified insights.
Accuracy and Model Training
AI-based systems depend on clean, well-labeled data to produce accurate results. Misinterpretation of tone or language nuances—especially in multilingual environments—can lead to false sentiment tagging or compliance errors. Ongoing model training and local language tuning are essential.
Change Management
Adopting CA platforms often requires cultural shifts in how teams evaluate performance. Managers and agents need to be trained not only in using the tools but also in trusting the insights. Resistance to automation or fear of surveillance can hinder adoption.
6 Best Practices for a Successful Conversation Analytics Strategy
To fully leverage the power of conversation analytics, organizations should adopt a strategic and scalable approach. The following best practices will help maximize your return on investment and ensure long-term success.
Define Clear Objectives
Start by identifying specific, measurable goals you want to achieve with conversation analytics. Common objectives include improving Customer Satisfaction (CSAT), reducing Average Handle Time (AHT), increasing First Contact Resolution (FCR), or ensuring compliance with regulatory standards. Clear goals provide direction for implementation and serve as benchmarks for performance evaluation.
Start Small and Scale
Instead of rolling out analytics across the entire contact center immediately, begin with a pilot program. Focus on one channel—such as voice or chat—or a single team to test effectiveness and uncover early insights. This allows for controlled testing, rapid iteration, and easier troubleshooting before scaling to additional teams or customer touchpoints.
Involve Key Stakeholders Early
Engaging stakeholders from the outset is critical. Include frontline agents, supervisors, QA managers, and compliance teams in the planning and decision-making process. Their input ensures the solution meets operational needs, and their involvement fosters better user adoption and cross-functional alignment during implementation.
Prioritize Data Quality
Accurate analytics depend on high-quality data inputs. Invest in reliable speech-to-text transcription, robust call metadata, and integration with your CRM or ticketing systems. Clean, consistent data is essential for generating accurate insights, building trust in the platform, and making informed decisions based on the analytics output.
Combine with Human Oversight
While AI provides speed and scale, human expertise is essential for contextual judgment. Use conversation analytics to complement—not replace—coaching, quality audits, and customer experience management. Supervisors and QA teams should interpret insights, provide tailored feedback to agents, and intervene in complex or sensitive situations as needed.
Monitor and Adjust Continuously
Post-implementation, regularly review performance metrics and user feedback. Track KPIs such as CSAT, resolution rate, and agent improvement over time. Be prepared to refine platform settings, retrain models, or adjust workflows based on evolving customer needs and business priorities. Continuous improvement ensures sustained ROI and long-term value.
Turn Customer Conversations into Actionable Insights with Nubitel AI
Conversation analytics empowers your contact center to move beyond traditional reporting by leveraging AI-driven insights for proactive decision-making. Whether you aim to improve agent performance, strengthen compliance, or better understand your customers, Nubitel’s conversation analytics technology delivers measurable business value.
Organizations that embrace Nubitel’s conversation analytics today will be better equipped to meet tomorrow’s service expectations—faster, smarter, and more customer-focused.
Nubitel AI Conversation Analytics platform equips service teams with real-time intelligence, multilingual support, and actionable insights, helping you optimize every interaction and gain a competitive edge.